You still need more exploration?
The cutting edge is here
Basic information
- Date & Time: 7th/March/2019 10:30--18:00
- Location: Institute of Industrial Science (IIS) in Komaba II Campus, Univ. Tokyo.
- 1st floor in Bldg. S (the former 60th bldg.)
- How to Access to IIS:
- Registration is required!!! (jump to registration form)
Programme
- 10:30-10:40: Opening Remark
- Session1: Understanding strategy of thermotaxis
- 10:40-11:20: Ikue Mori from Nagoa Univ., Japan
- Exploitation and exploration modes in a C. elegans navigation behavior
- 11:20-11:50: Naoki Honda from Kyoto Univ., Japan
- Identification of animal behavioral strategies by inverse reinforcement learning
Lunch
- Session2: Biological sensing & searching strategies
- 13:00-13:30: Akihito Nakajima from Univ. Tokyo, Japan
- Relationship between cell polarity dynamics and search strategy in amoeboid migration
- 13:30-14:00: Shizuko Hiryu from Doshisha Univ., Japan
- Learning from biosonar system - laboratory and field studies on acoustic navigation of bats
Short break
- Session3: The cutting-edge of learning theory
- 14:30-15:00: Taiji Suzuki from Univ. Tokyo, Japan
- Compressing deep neural network and its generalization error analysis via kernel theory
- 15:00-15:30: Eiji Uchibe from ATR
- Imitation learning under entropy regularization
Break
- Session4: Potential applicability of RL for biological intelligence I
- 16:00-16:30: Kenji Morita from Univ. Tokyo, Japan
- Neural mechanisms for reinforcement learning and motivation
- 16:30-17:00: Tetsuya J. Kobayashi from Univ. Tokyo, Japan
- Understanding adaptive immunity as a reinforcement learning system
Short break
- Session5: Potential applicability of RL for biological intelligence II
- 17:15-17:55: Antonio Celani from ICTP, Itally
- Learning to navigate in dynamic environments
- 17:55-18:00: Closing Remak
Speakers' information & abstract
Ikue Mori
- Professor of Group of Molecular Neurobiology
- Department of Molecular Biology, Graduate School of Science, Nagoya University
TBA
Naoki Honda
- Associate Professor of Lab of Theoretical Biology
- Graduate School of Biostudies, Kyoto University
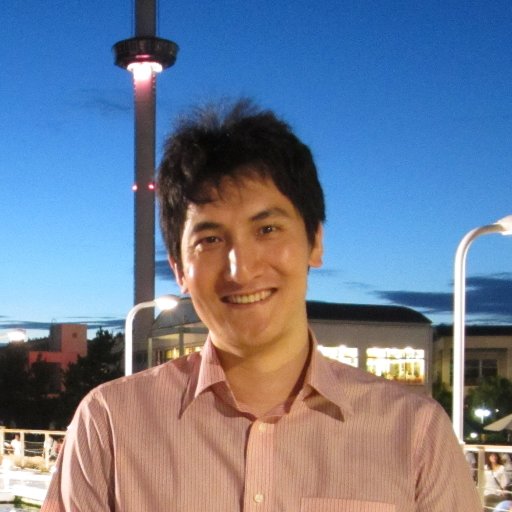
Understanding animal decision-making has been a fundamental problem in neuroscience. Many studies have analyzed the actions representing decision-making in behavioral tasks involving artificially-designed rewards with specific objectives. However, it is impossible to extend such experiment to a natural environment in which the rewards for freely-behaving animals cannot be clearly defined. In this talk, we present a new computational approach (inverse reinforcement learning), which reverse the current paradigm so that rewards could be identified from behavioral data from time-series data of freely-behaving animals. By applying this technique on C. elegans thermotaxis, we successfully identified the respective reward-based behavioral strategy.
Akihiko Nakajima
- Assistant professor of lab of biophysics (link)
- Department of General Systems Studies, Graduate School of Arts & Sciences, University of Tokyo
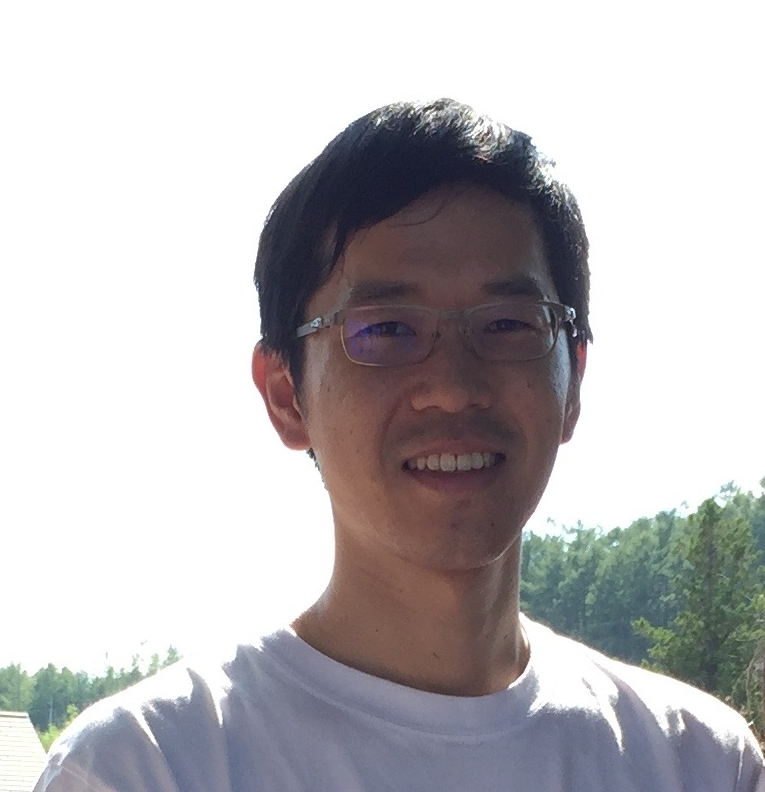
Amoeboid cells have amazing adaptive capacity to recognize changes in the complex environments and migrate accurately to a target place. Using immune cells and Dictyostelium amoeba as a model system, we have studied how such ability is realized from a combined approach of fluorescent live-cell imaging, microfabrication, and nonlinear physics. In my talk, I will show how different modes of migration and sensing ability are emerged from dynamical systems properties such as reaction-diffusion characteristics inherent in intracellular chemicalreaction and discuss the relationship between such properties and behavioral strategies of migrating cells.
Shizuko Hiryu
- Professor of Neuroethology and Bioengineering Lab.
- Graduate School of Life and Medical Sciences, Doshisha University

Bats possess a highly developed SONAR system. To learn their acoustic navigation strategies, we conducted several behavioral studies from small scale in a laboratory to large scale navigation in the field. First, I will present jamming avoidance behavior of bats during group flight. Using on-board microphone technique, we found that group members broaden the inter-individual differences in the frequencies of emitted ultrasounds to decrease the similarity of sensing signals among individuals. I also introduce the navigation behavior of wild bats during natural foraging. Through various behavioral measurement, we would like to approach the behavioral principles and information processing mechanism that support their advanced acoustic navigation.
Taiji Suzuki
- Associate Professor (personal HP)
- Department of Mathematical Informatics, University of Tokyo
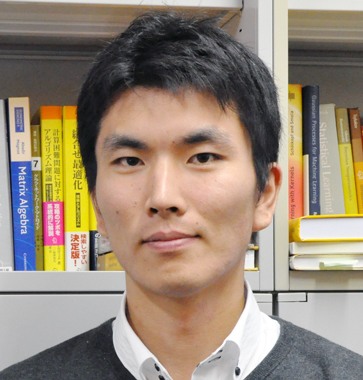
In this talk, we discuss the generalization error of deep learning by analyzing the complexity of deep neural network models in some settings. We define a data dependent intrinsic dimensionality of deep neural network models and show how it affects generalization performance. To analyze that, we define a reproducing kernel Hilbert space for each internal layer, and borrow theoretical techniques from kernel methods. It gives a fast learning rate which has mild dependence on the network size unlike the previous analysis. We also develop a simple compression algorithm which is applicable to wide range of network models, and show that it gives preferable compression performance.
Eiji Uchibe
- Principal Researcher (personal HP)
- Dept. of Brain Robot Interface, ATR Computational Neuroscience Laboratories
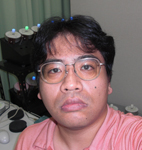
We proposes Entropy-Regularized Imitation Learning (ERIL) that is given by a combination of forward and inverse reinforcement learning under the entropy-regularized Markov decision processes. Inverse RL is interpreted as estimating the log-ratio between the expert and the baseline policies, which is efficiently solved by binary logistic regression. Forward RL is a variant of Soft Actor-Critic that minimizes the KL divergence between the learning and the estimated expert policies. Experimental results on some benchmark tasks show that ERIL is more sample efficient than the previous methods because the forward RL step of ERIL is off-policy.
Kenji Morita
- Associate Professor (personal HP)
- Physical and Health Education, Graduate School of Education, The University of Tokyo
Impairments of the signaling of neurotransmitter dopamine have been shown to cause motivational impairments, such as shifts in the preference from high-cost-high-benefit to low-cost-low-benefit options. Meanwhile, the activity pattern of midbrain dopamine neurons was found to resemble the pattern of the temporal difference (TD) error defined in reinforcement learning algorithms, and dopamine-dependent synaptic plasticity has been suggested to implement prediction error-based update of state or action values. However, neural circuit mechanisms for the calculation of the TD error, as well as mechanistic bases of the motivational impairments, remain elusive. I introduce my own and others' recent work on these issues.
Tetsuya J. Kobayashi
- Associate Professor of Lab of Quantitative Biology
- Institute of Industrial Science, University of Tokyo
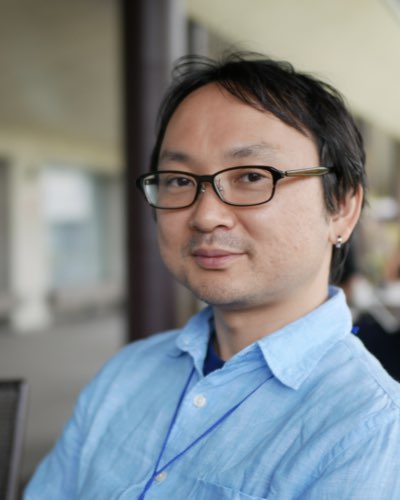
Adaptive immunity is responsible for recognising and detecting various pathogens never being inexperienced and for learning signatures of the new pathogens as well as how they should be handled. The goal of the adaptive immunity and the way how it conducts learning are entirely consistent with the framework of reinforcement learning. In this talk, we present how we can frame the process of adaptive immunity into reinforcement learning, and show well-known learning rule of the adaptive immunity can be derived from this framework. We also touch on the properties achieved after convergence of learning and compare those with experimental data.
Antonio Celani
- Research associate of Group of Quantitative Life Sciences
- Abdus Salam International Centre for Theoretical Physics
Soaring birds often rely on ascending air currents as they search for prey or migrate across large distances. The landscape of convective currents is rugged and rapidly changing. How soaring birds find and navigate thermals within this complex landscape is unknown. Reinforcement learning provides an appropriate framework to identify an effective navigational strategy as a sequence of decisions taken in response to environmental cues. I will discuss how to use it to train gliders to autonomously navigate atmospheric thermals, in silico and in the field.